Trust: The key to AI adoption in the asset management sector
By Chrystelle Veeckmans; Benedikt Höck, KPMG
Published: 23 September 2024
Asset managers are keen to unlock the potential benefits of generative AI. But a lack of trust is getting in the way. Here’s how the most advanced asset managers are building a foundation of trust for AI and driving adoption and value in the process.
If you are like most asset management executives, you’ve probably been spending quite a bit of time talking about generative AI. You’ve likely seen dozens of potential use cases in the asset management space. And you are already well aware that ‘gen AI’ will soon become embedded right across the asset management value chain. Chances are, you see generative AI as a potential competitive advantage rather than a risk. But are you moving fast enough to seize that advantage?
According to a survey conducted in 2023 by KPMG in the US, 74% of executives think generative AI will be the top emerging tech to impact their business over the next year and a half.1 And a global survey of CEOs shows that 70% are investing heavily in generative AI as their competitive edge for the future, with most (52%) expecting to see a return on their investment in three to five years.2
Yet progress adopting generative AI in the Asset Management space has been slow when compared to other sectors – even within the financial services industry. Some are now testing embedded AI features such as Microsoft’s Copilot tools. Others are implementing platforms that allow employees to train their own assistants or to automate non-core activities (like office scheduling). Indeed, our work with leading asset managers suggests that the vast majority are still in the exploration phase.
What is slowing progress?
We believe it is trust – or a lack thereof. A lack of trust undermines confidence. It discourages exploration. It limits ambition. It creates massive challenges right across the value chain. And the root causes of the lack of trust are manifold.
In part, the lack of trust can be chalked up to the speed at which generative AI has burst onto the business scene. Within just 18 months, it has become mainstream. That’s faster than many asset managers turn around an equity holding. And it’s not a lot of time for managers to build up trust. They’ve seen edge cases where models didn’t react as anticipated. Most would rather wait until the use cases are 100% reliable before they even consider embedding them into their businesses.
Many asset managers (rightly or wrongly) distrust the nature of the generative AI beast. Transparency is hard to find – the training data and models are often owned and managed by a third party (often Big Tech); the decision-making process is generally unexplainable (however correct the outcome may be); few, if any, people in the business really understand how this stuff works.
Then there are the use risks that keep asset managers up at night. They worry that their employees might use the technology in the wrong way, wrong context or wrong process, thereby creating reputational, financial and trading risks. They are concerned that their own internal data may not be reliable, leading to poor decisions. They agonise about the impact of future regulation, particularly as the EU member states move to adopt the EU AI Act and integrate it into their financial regulation systems.
Building trust
The key to driving generative AI adoption, value and innovation in the asset management sector, therefore, is trust. We believe those who are able to develop a foundation of trusted AI and then scale up will be the ones who are best placed to reap its competitive advantages. Those who leave trust as an afterthought will likely struggle to embrace AI and manage the related risks and challenges.
At KPMG, our experience reveals five key areas where asset managers will want to focus in order to better build trust in their AI.
- Strategy. The leaders have a clarity of vision about AI that unites their organisation and builds confidence about the future direction. The frontrunners have a strategy in place, supported by a clear story about how AI supports the overarching goals of the company. They have redesigned their operating models to reflect key aspects of AI – including things like technology impact, workforce optimisation and governance and controls. They understand the business priorities driving the adoption of AI and are aligning their technology spend and effort accordingly. And they are adapting and evolving their approach as their experience and AI capabilities grow.
- Governance. The leading asset managers are currently focused on ensuring that good governance is embedded as part of their AI strategy and target operating models. Those in the EU, in particular, are starting to implement measures to respond to key aspects of the EU AI Act, such as creating an inventory of use cases and related risk classifications. While governance should ensure that responsibility for AI flows from the very top of the organisation, great care must be taken to also implement controls and governance at an individual level across the organisation. Generative AI is rapidly spreading across the organisation – all three lines of risk management defence must be ready.
- Data and models. There are two key inputs to every AI solution – the data and the models. Both must be trustworthy and reliable. With public Large Language Models (like ChatGPT, for example) it is often challenging to assess the quality of the underlying training data and foundational models. We are seeing a number of asset managers start to explore whether they can create their own smaller language models, particularly for higher risk applications, based on their own data. Those with a pre-existing data strategy and structured data layer are generally starting off from a better position; those without are struggling to catch up quickly.
- People. Getting your people comfortable with AI and helping them understand the risks and opportunities is key to driving trust within the workforce (we are working with one asset manager to deliver ‘AI boot camps’ for new managers, for example). Leading firms are creating safe spaces for employees to test ideas and become familiar with new AI tools and technologies rather than banning them completely. At the same time, it is also important to assess the workforce implications and to communicate the impacts clearly, particularly where people’s roles may be impacted. As a result, we are seeing the leading asset managers ramp up their change, enablement, communications and development programs related to AI.
- Security. In the KPMG 2023 CEO Outlook, 82% of leaders globally said they were worried that AI may provide new attack strategies for adversaries. And we are already seeing a number of new attack strategies emerge – like, for example, ‘prompt injections’ where hackers feed the AI malicious prompts in order to fool the system into revealing sensitive information like customer accounts. The leading asset managers are reassessing their cyber defence and resilience strategies and capabilities in order to address and manage the new risks, vectors and tactics enabled by generative AI.
To find out more and to benefit from our experience, we encourage you to contact us to discuss your unique objectives.
1. 2023 KPMG Generative AI Survey, KPMG LLP
2. KPMG 2023 CEO Outlook, KPMG International
“Generative AI is spinning out a range of exciting use cases for the Asset Management sector. Those able to selectively integrate these ideas and tools into their operations and trading will almost certainly enjoy a competitive advantage – but only if they put trust at the centre of their strategic decision-making.”
Andrew Weir, Regional Senior Partner, KPMG in Hong Kong (SAR); Vice Chairman, KPMG China; and Global Chair, Asset Management and Real Estate, KPMG International
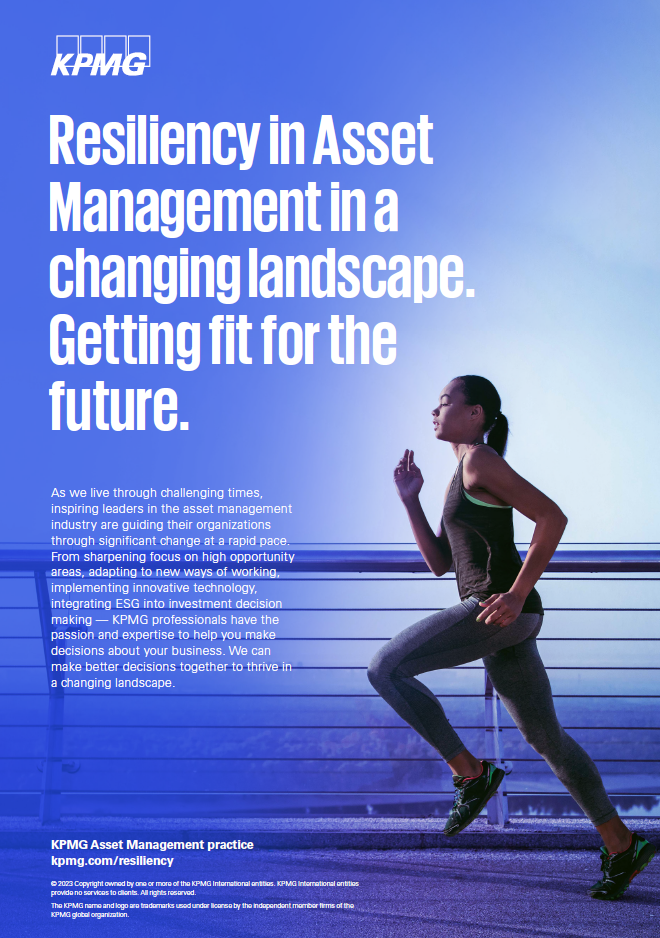