Leveraging data and AI for innovation in asset management
By Carl Barrelet, Head of Data Science, KPMG in Canada
Published: 28 June 2021
The importance of utilising data and AI models for the better screening and monitoring of investments and risks has grown steadily across the asset management industry in recent years. It is fair to say, though, that through the COVID-19 pandemic, its significance has never been higher. The unprecedented levels of volatility and unpredictability that the outbreak introduced into the investment landscape means the ability to predict and manage risks, especially on the downside, has become more critical than ever.
Those asset managers that have a dynamic handle on risks and a more sophisticated view of the impacts of the pandemic on both portfolios and individual stocks will have a significant advantage over their peers. We have already seen a growth in interest in ‘COVID datasets’ from alternative data vendors for example, offering information on previously largely ignored data such as patient numbers and hospital admissions in cities and regions, as well as real-time foot traffic in cities around the world as an indicator of economic activity.
To a large extent, advanced data and AI tools used to be concentrated in the hands of a limited group – top tier funds and hedge funds with deep pockets – but as data has become more accessible across organisations, and as more powerful models have become available for investors, it’s now much more feasible for other players to create and run live data models. It is a trend that has become increasingly pronounced across the sector – and one that we can expect to continue to grow strongly, especially as firms navigate what will most likely prove to be a gradual and stop-start recovery period through this year. has become more critical than ever.
Getting the operating model on target
But what are the factors for success? It’s critically important that asset managers devise the right target operating model (TOM) for their data approach. There is no single operating model that works for all firms – it’s a case of aligning the approach with the organisation’s capabilities, objectives, culture and maturity with respect to producing and delivering innovation.
It is also the case that different parts of a firm may be more in tune already with data and AI than others. The systematic trading side, for example, are often advanced already in leveraging aspects of artificial intelligence (AI) and machine learning (ML), while other parts of an organisation remain more relationship than data-driven, closer to the traditional private equity or venture capital model. It will also vary from portfolio manager to portfolio manager – some will be enthusiastic while others may be skeptical, so taking account of the receptiveness of individual PMs is important.
Operating models for data and innovation can range widely from highly centralised (all data scientists co-located in one team, separate from the rest of the business) right through to decentralised and independent (data scientists integrated directly into teams across the organisation). The truth is that the most successful models are likely to be somewhere in between – a hybrid approach. The danger of a very centralised approach is that the data team is too removed from the portfolio managers who are making investment decisions on the ground; while a highly decentralised model can mean duplication of effort and inefficiency.
The choice of operating model will have implications for aligning with operational functions such as data management, technology architecture and resources, and data & model governance.
It’s also key to appreciate that an organisation’s operating model is likely to evolve over time. It may look quite different in year three, for example, to how it looked in year one. Most likely, it will start off relatively centralised and then move further to the right over time.
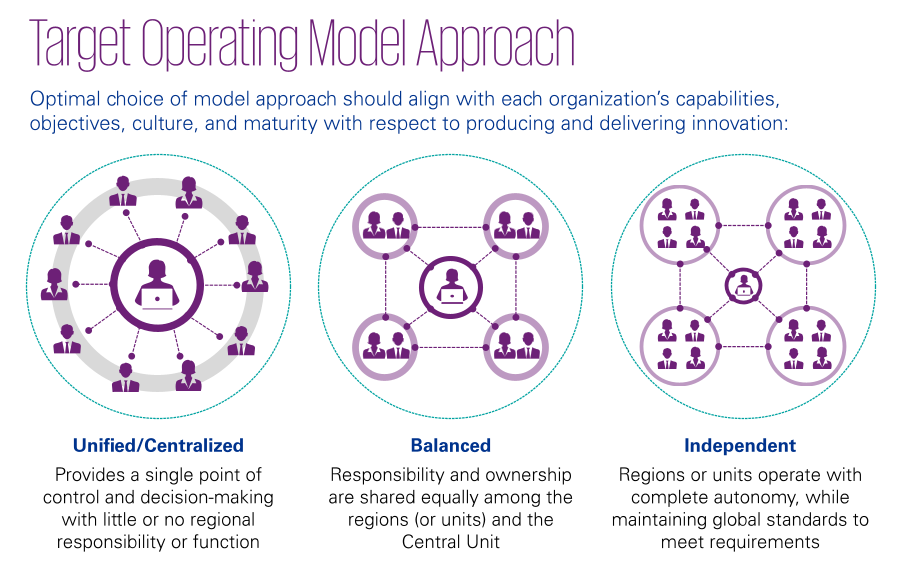
Getting your TOM right is an essential first step to properly leveraging data and AI across the enterprise. It’s vital that you get the right people round the table (or, the virtual table) to have a full and considered discussion, and recognise that it will be a journey not a one-off transformation. The ultimate goal must be to reach the position where emerging technologies enable the organisation to use data not as cost to be managed but as a profit center – creating alpha generation from AI insights.
Implementing AI tools for real results
But how should an asset manager utilise these high-potential tools? Again, there are various possible models. A portfolio manager may simply read a report that has been generated through the application of data and AI and then make decisions accordingly; equally, a data signal may feed directly into an algo-trading model itself.
Models may be developed in-house, or they may be taken from a provider. Or there may be a hybrid approach – working together to develop and enhance a model that can then be applied across a portfolio.
Today’s models are becoming increasingly sophisticated, making use of a range of technologies such as AI, ML and natural language processing (NLP). They don’t stand still either – nowadays, there is not only ML and NLP but advanced variants such as computer vision, language models and reinforcement learning Data-driven solutions are commonly used across three main areas. Some are for screening investments and forecasting merger and acquisition (M&A) events ahead of time. M&A plays a critical role in the lifecycle of an investment – understanding the likelihood of an M&A event gives powerful insights to screen investments. Firms are able to identify and rank companies according to the likelihood of their becoming a target and optimise their investment approach as a result – allowing them to take advantage of the model’s prediction scores before it becomes too late to react.
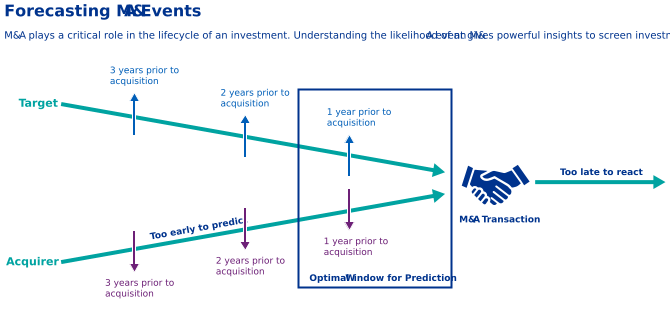
Other tools help to monitor investments and forecast default risks ahead of them coming to pass. Early prediction of default can empower investors to make well-informed and timely decisions. For example, a default predictor developed at KPMG Lighthouse ingests about 20 million data points spanning the previous 10 years, covering all public companies across North America. The model can be reviewed, validated and tuned across hundreds of iterations to achieve optimal performance. The output also includes explanatory detail.
Another important use case is leveraging natural language processing to consistently read the news generated globally by the news provider to pick up potential risk and reputational issues – data and AI techniques provide a powerful tool. Common risk areas include ethics violations, fraudulent activities, safety issues and external factors such as acquisitions or associations with third parties. Particularly where social media is involved, reputation-altering events can arise and take hold extremely quickly so having a real-time monitoring dashboard powered by advanced technologies could help to significantly reduce the financial or social impact of adverse experiences and events.
It is important to remember, however, that these tools are intended to augment human judgment, not replace it. They provide insights that would otherwise be difficult (or impossible) for a human being to capture – but ultimately, it remains with the portfolio manager to make final decisions. Used in the right way, though, they can provide invaluable assistance that make a PM’s job easier, faster and much more data-driven: they take old-fashioned investment manager intuition into new, data-driven grounded realms.
The battle for skills and people
Another key factor for success is a difficult one to crack: obtaining the right talent to build the models. To leverage data and AI, firms need a new breed of people. Whereas in the past, intakes used to be based around attracting individuals with a heavy focus on advanced financial or accountancy skills now firms are just as likely to want to bring in people who can code in Python or other advanced programming languages.
This is a significant shift. The need for the ‘old’ skills has certainly not disappeared, but the requirement for new technology-based skills has rapidly shot up the agenda. Competition is high and the big tech giants tend to have the upper hand. They can afford to pay big salaries and have the cache and reputation for cutting-edge innovation that naturally attracts the people (often Millennials) with the highly specialised skills needed.
However, the investment industry also holds significant attractions so firms should certainly not lose heart. With such strong correlations between investment strategy and data, many highly talented software engineers, machine learning engineers and data scientists could find a fulfilling and rewarding career at a progressive asset manager.
Remember also that it doesn’t come down to getting that one, perfect person: it’s about assembling a team with complementary skills across it. Working with external organisations can also be a route to success.
2020 was a huge test for many in the industry; 2021 continues to be challenging, given the uncertainties over the pace and breadth of economic stabilisation and recovery. Whatever the future brings, embedding effective models for data-driven innovation will be a key determinant in asset managers’ performance.